量子ニューラルネットワーク(QNN)
量子とAIの融合を切り拓く
量子ニューラルネットワーク(QNN)は、量子機械学習の基礎であるニューラルネットワーク理論と量子コンピューティングの最先端の融合を体現している。重ね合わせやエンタングルメントのような量子原理を利用することで、QNNは従来のニューラルネットワークの能力を大幅に増強します。これらの高度なネットワークは、大規模で複雑なデータセットを扱うことに長けており、複雑なパターン認識やデータ集約的なタスクに理想的である。
量子コンピューティングとAIの融合
QNNは、量子コンピューティングのブレークスルーを人工知能や機械学習と融合させるという願望から発展してきた。この融合は、量子特性を利用してニューラルネットワークの学習能力と処理能力を増強することを目的としている。量子機械学習で急成長している研究分野として、QNNは複雑な計算タスクのために量子力学のユニークな特性を利用することに焦点を当てている。
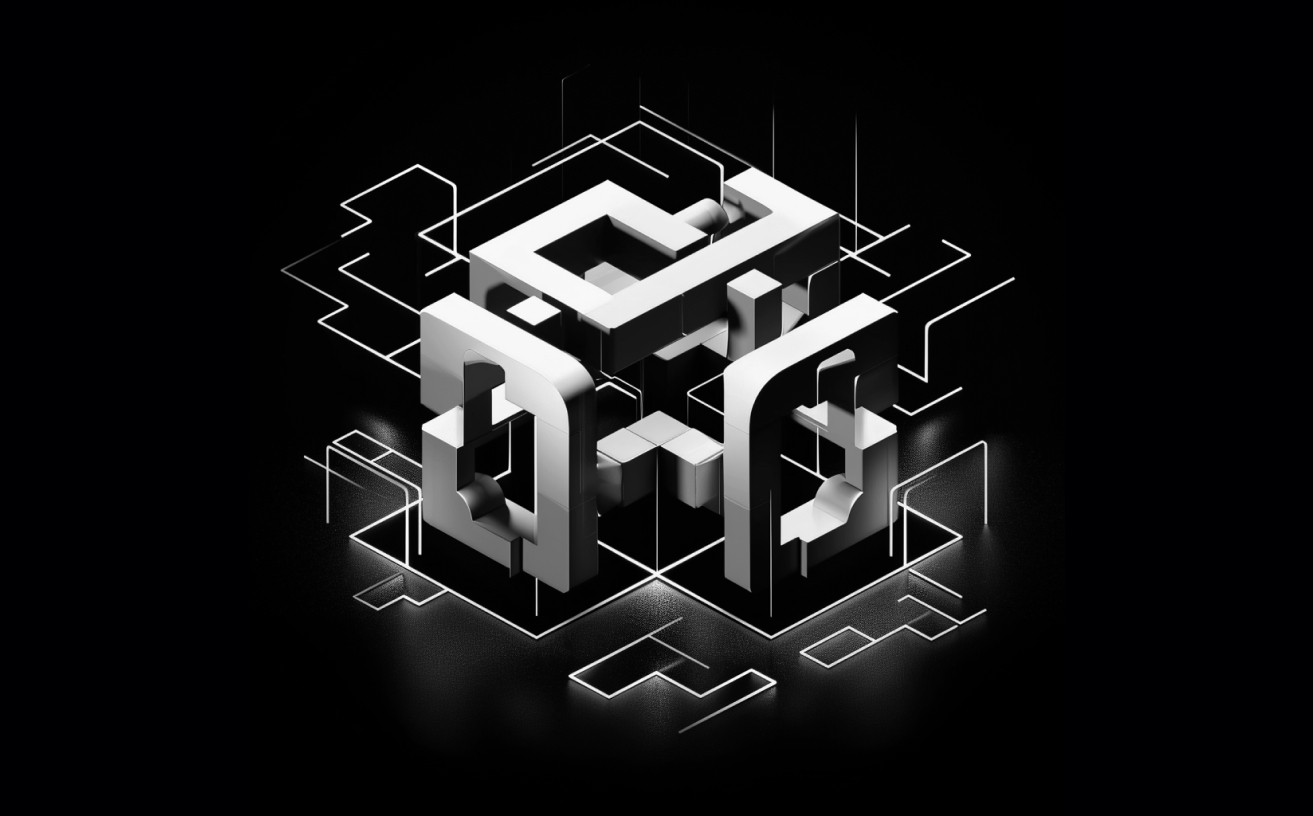
QNNメカニクスとアーキテクチャ
Classiqのフレームワークでは、QNNは古典的なものと同様にレイヤーごとに構造化されているが、量子的なひねりが加えられている。各層は、量子データ上で動作するパラメータ化された量子ゲートで構成され、オーダーメイドのQNNアーキテクチャを作成することができる。QNNは量子データの処理に長けており、量子状態の分類や他の量子中心のタスクに適しています。
QNNの学習プロセスは古典的なネットワークと同様で、量子ゲートのパラメータを最適化することに重点を置いている。この最適化には、多くの場合、量子と古典のハイブリッド・アルゴリズムが用いられ、両方のコンピューティングの長所を融合させることで、学習効果を高めている。
QNNの多様な応用と進歩
Classiqのプラットフォーム上に実装されたQNNは、特に量子データ処理や複雑な問題解決など、様々な領域で応用されています:
量子状態の分類と処理QNNは、量子コンピューティングや情報理論において重要な量子状態の分類と処理に優れている。
量子データ・エンコーディング:量子力学を利用してデータを符号化し処理することで、特定のデータ型に対してより効率的なアルゴリズムを提供できる可能性がある。
量子と古典のハイブリッド機械学習:量子データ処理と古典データ処理を組み合わせたタスクにおいて、QNNは革新的な問題解決アプローチを提供し、機械学習の範囲を拡大する。
さらに、QNNはいくつかの分野で有望な可能性を秘めている:
複雑なデータ処理:従来のニューラルネットワークが計算量の制約に直面するような膨大なデータセットを扱うのに特に効果的である。
量子化学:QNNは、量子効果が最も重要な領域である分子や原子の相互作用のシミュレーションに非常に有効です。
高度なパターン認識:QNNはその強化された能力により、高次元データのパターン認識に優れており、画像認識や音声認識などの分野に応用できる。
生物医学への応用:創薬やゲノム解析において、QNNは複雑な生物学的データの処理に革命を起こす可能性を秘めており、複雑な生物学的システムに対する洞察を提供し、医学のブレークスルーを助ける。
AIと量子コンピューティングの融合:Classiqで量子ニューラルネットワークを発見する!
プラットフォームについて https://docs.classiq.io/latest/user-guide/built-in-algorithms/qml/qnn/qnn/
"キュービット・ガイのポッドキャスト "について
The Qubit Guy(弊社最高マーケティング責任者ユヴァル・ボーガー)がホストを務めるこのポッドキャストは、量子コンピューティングのオピニオンリーダーをゲストに迎え、量子コンピューティングのエコシステムに影響を与えるビジネスや技術的な疑問について議論します。ゲストは、量子コンピュータのソフトウェアやアルゴリズム、量子コンピュータのハードウェア、量子コンピューティングの主要なアプリケーション、量子産業の市場調査などについて興味深い見解を提供します。
ポッドキャストへのゲスト推薦をご希望の方は、こちらまでご連絡ください。